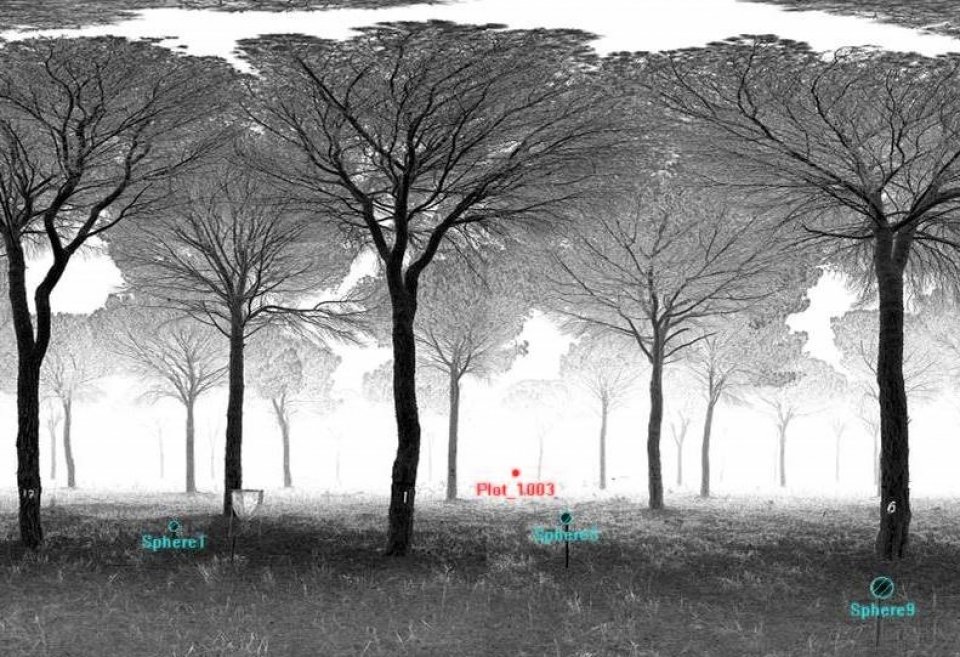
The objective of the present study is to better understand the relationship between tree characteristics and cone production of Mediterranean stone pine. This was achieved by quantifying the gain in using detailed crown metrics in estimating cone production at individual tree level (number of cones per tree and average cone weight). Models based on traditional variables (tree size and stand characteristics) were compared to models that relied on crown metrics extracted from TLS data. The resulting models should help owners and managers to better predict cone production.
The pine nut kernels in the cones of Pinus pinea are forest nuts of great economic value. Cone yield harvesting rights are often sold through annual leases, the price of which depends on the expected yield which varies greatly between years (masting, cf. Calama et al. 2016). Visually evaluating cone production on standing trees is challenging since the cones are formed in the upper part of the crown. Hence, yield estimation is commonly performed out by using predictive models. This study evaluates how new remote sensing technologies such as terrestrial laser scanners (TLS) can be used to assess cone productivity, or to attempt to explain the tree-to-tree variability within a given stand.
Tree size, competition, stand density and maturity, and site conditions influence cone production of a tree. However, there are differences in production between trees of similar status and dimensions in the same stand. Therefore more detailed crown and microsite information obtained by TLS were explored to better understand the between-tree variations.
Using data from 129 trees in 26 plots in Spain, models with TLS metrics showed better fit statistics than models solely based on traditional inventory data for predicting the cone yield of individual trees. Crown dimensions such as projected crown area and crown volume, crown density and crown asymmetry were the key TLS-derived drivers for the variability in inter-tree cone production.
The number of cones per tree was determined by both crown volume and density. Both estimates, from ground or TLS-based, show that the larger the crown the more cones a tree bears. TLS captures irregularities and asymmetries in the crown shape going beyond the simplified crown form assumed from ground. However, the number and size of cones decrease with crown density. Stone pine crown shape varies with tree development, as the spherical crown of young trees with foliage on the whole external surface shifts toward a smoothly rounded umbrella-like shape with foliage only covering the upper, fully sun-exposed part of the crown.
The links between crown dimensions and cone production show managers the relevance of favouring growing conditions that enable the trees to develop large, symmetrical crowns. Crown pruning might also be considered to increase cone production.
Models based on new remote sensing technologies (TLS) clearly outperformed inventory-based models. The results indicate that crown dimensions are important for understanding cone production in stone pine. Our work points to the potential of TLS as a tool for rapid, accurate, and unbiased measurements of crown parameters not easily accessible from the ground.
Although obtaining TLS measurements in the field is relatively rapid, there is still a need to develop easy-to-use algorithms to process the data that must otherwise be processed manually.
The TLS attributes that were found to be important, viz. crown volume and density, or terrain roughness, can also be derived from other remote sensing technologies such as aerial laser scanner or drone-based sensors that might be useful in estimating cone productivity on an operational scale.
Quick, large-area estimates of cone production could ultimately be achieved and help managers identify areas of high cone productivity. Thus, such sensors might offer detailed cone productivity maps at reduced costs.
RafaeL Calama, rcalama@inia.es, www.inia.es
Robert Schneider, robert_schneider@uqar.ca, https://www.uqar.ca
Further information
Schneider R, Calama R, Martin-Ducup O (2020). Understanding Tree-to-Tree Variations in Stone Pine (Pinus pinea L.) Cone Production Using Terrestrial Laser Scanner. Remote Sensing 12: 173. doi:10.3390/rs12010173
(c) R. Schneider
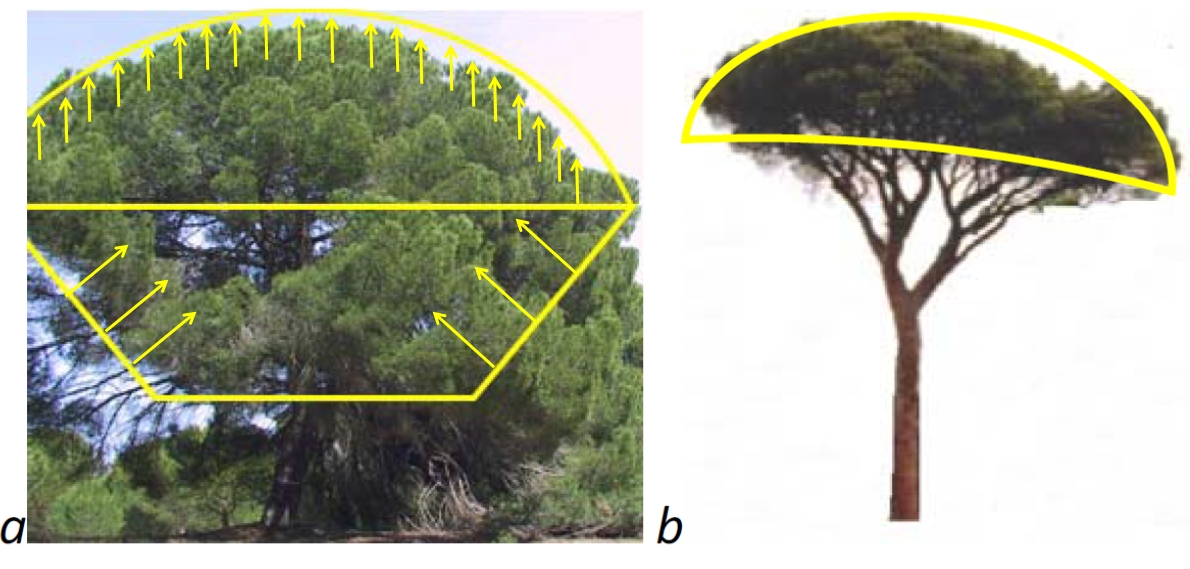
Crown development in stone pine (a. adult tree with expanding crown, but receding inner/lower crown; b. aged tree with hollow crown)
(c) S. Mutke